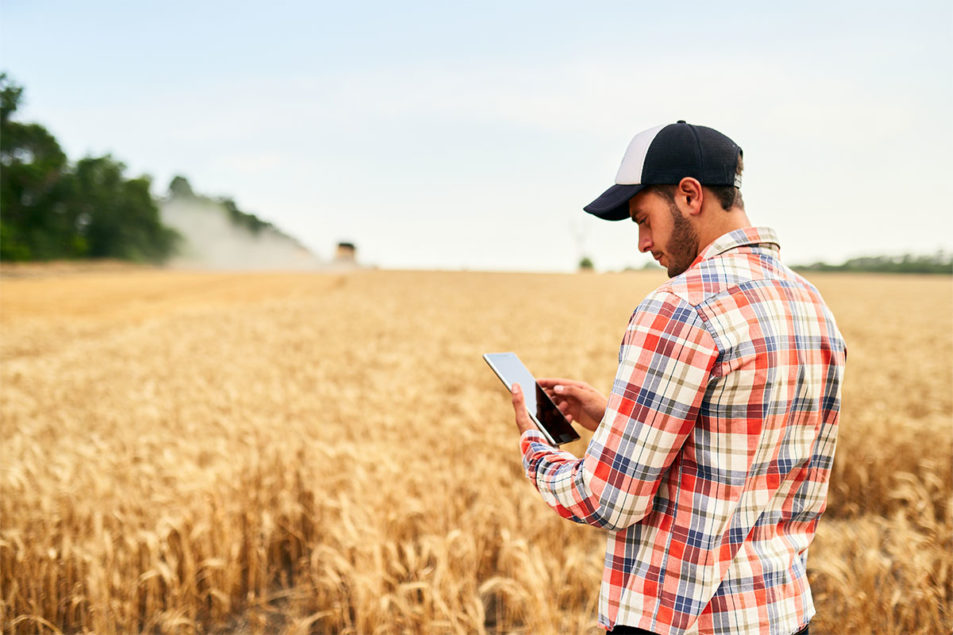
WASHINGTON โ Artificial intelligence stands as the fourth revolution in agriculture, โwhich is truly expected to transform agriculture,โ said Madhu Khanna, PhD, professor; director of iSEE; ACES Distinguished Professor in Environmental Economics at the University of Illinois, Urbana-Champaign, at the 100th annual US Department of Agriculture Agricultural Outlook Forum.
A diverse array of AI applications in agriculture is currently under exploration within universities and research institutions worldwide. These advancements were discussed extensively by Khanna and others at the forumโs Feb. 15 session titled โRobotics and AI for Sustainable, Equitable Agricultural Systems.โ
One of the most promising avenues for AI lies in its ability to harness machine learning techniques for analyzing data sourced from precision farming, GPS, maps, and other relevant datasets, the presenters said. However, as Dr. Khanna notes, a salient question remains: โHow can we use this data to make better decisions and to reduce the uncertainty of farming in order to increase the predictability and recommendations we can make that would really provide benefits to the farmer?โ
Dr. Khanna also addressed the challenges associated with transitioning decision-making processes to AI. Farmers often have multifaceted objectives guiding their decisions, making it challenging to replicate this nuanced decision-making within AI systems.
Moreover, there exists a notable reluctance, or โalgorithm aversion,โ among individuals to cede control to automated systems, mirroring the apprehensions seen in other domains, Khanna said.
โHanding over the charge for how to manage a farm to a robot is going to take some level of adaptation,โ Khanna said. โMany of us would be very reluctant to let a robot make investment decisions over our life savings instead of having a financial adviser and thatโs the kind of thing you can imagine for a farmer would be very similar.โ
To mitigate uncertainties in farming outcomes, Khanna advocated for an overhaul in farming data accessibility, as existing contractual arrangements between farmers and implementing companies may impede useful data from becoming available to developers and companies that would enhance AI technologiesโ predictive capabilities.
โThere are very strict rules on who owns the data, and what it can be used for,โ she said. โSo under current rules, a lot of the data collected from farmersโ fields can only be used to benefit the farmer. And companies are limited in terms of their ability to compile data from multiple farmers and then use it for general improvement in technology. So we need to move considerably far from that arrangement in order to be able to use and improve these technologies. The greater amount of data available, the better these technologies are able to predict and learn from that. So with AI technologies, having data networks is going to be really important.โ
Khannaโs focus on increasing accessibility to data resonated with comments from panelist Ethan Rublee, founder and CEO of the robotics company farm-ng.
Rublee explained how AI systems like ChatGPT benefit from an abundance of data to train on, but farming does not yet have that advantage, he said, emphasizing the need for improved data logistics. He also highlighted the need for labeled data to be able to train AI models to understand complex ag robotics systems.
โI think in this 10-year horizon affecting us is getting improved modeling of these really complex systems,โ Rublee said.
Meanwhile, another important application for agricultural AI resides in livestock farming, where technologies are being researched and developed by the Artificial Intelligence for Future Agricultural Resilience, Management, and Sustainability (AIFARMS) Institute, where Khanna is associate director of technology adoption and public policy. Researchers at AIFarms developed โcomputer visionโ to monitor animals around the clock, track their overall health, the status of their reproductive cycle, and maintain facilities with minimal human labor.